Business
Unlocking Business Value with Data Science
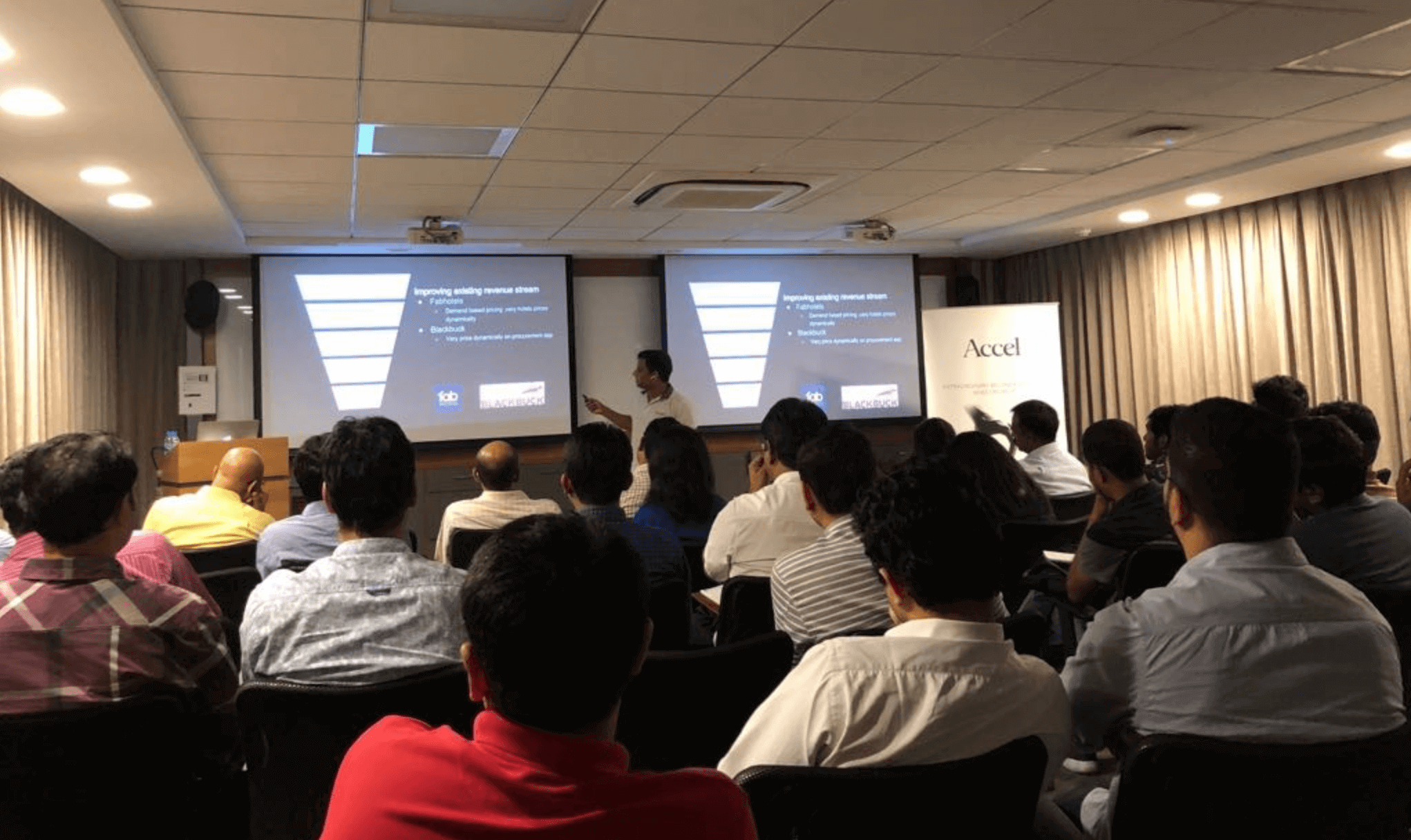
Summary
The advent of data science, machine learning, and artificial intelligence has gained visibility across platforms such as conferences and social media. Nonetheless, a large proportion of startup founders face challenges when identifying key areas where businesses would benefit from using data science. 80% of a data scientist’s time is devoted solely to finding, filtering, and organising data, leaving only 20% to actually perform data analysis. Data collection and storage are crucial requisites for the success of any project in the domain of data science.
Why does data science matter and how does it power business values?
To answer this, Accel organised the event ‘Unlocking Business Value with Data Science,’ where founders and data leaders from the Accel family came together with data product experts, who expounded the benefits of data. Guest speakers included:
- Swaminathan Padmanabhan — Director of Data Science at Freshworks
- Ambarish Kenghe — Leading Tez Product Management at Google, Former Chief Product Officer at Myntra
- Sharath Bulusu — Senior Vice President of Product Management at Myntra
Through this blog post we will be discussing the following key points of using data science at Startups.
- The concrete benefits that Accel portfolio companies perceive in using data science today.
- Data collection and data storage as key pillars for the resolution of data science problems. Data quality and volume are crucial; “garbage-in-garbage-out” applies across all data science projects.
- The benefits of simple data science techniques such as exploratory data analysis, regression, and decision trees. Almost 70% to 80% of business problems can be resolved using simple techniques if the required data is available for processing. 90% of the data science cases explored during this session were resolved using straightforward methods.
In order to prepare for the data-driven world, Accel recommends that start-ups:
- Learn to collect data in the correct format (minor lapses could result in a delay of 6 months)
- Effectively organise data for analysis (termed ‘data pipelines’)
- Cultivate a curiosity to explore
The event commenced with sharing data-driven case studies related to Accel portfolio companies. Of the 120 portfolio companies, at least 30 companies are using data to derive business insights and to add value to their businesses. These insights help build data products in the fields of fraud prevention, personalised customer experiences, increased sales, streamlined operations, and developed customer engagement. The insights also empower management to take better decisions using data. While working with Accel portfolio companies, it was observed that almost 80% of business problems were solved with basic data science techniques (such as regression, binary classification, tree based models, etc.).For solving key business problems you don’t really need to jump to neural network. You might rather identify what problems can be solved by analysing data you have collected over the period of running business operations.
To understand customer behaviour and their engagement with the platform ,data science can be applied at each step of Funnel.
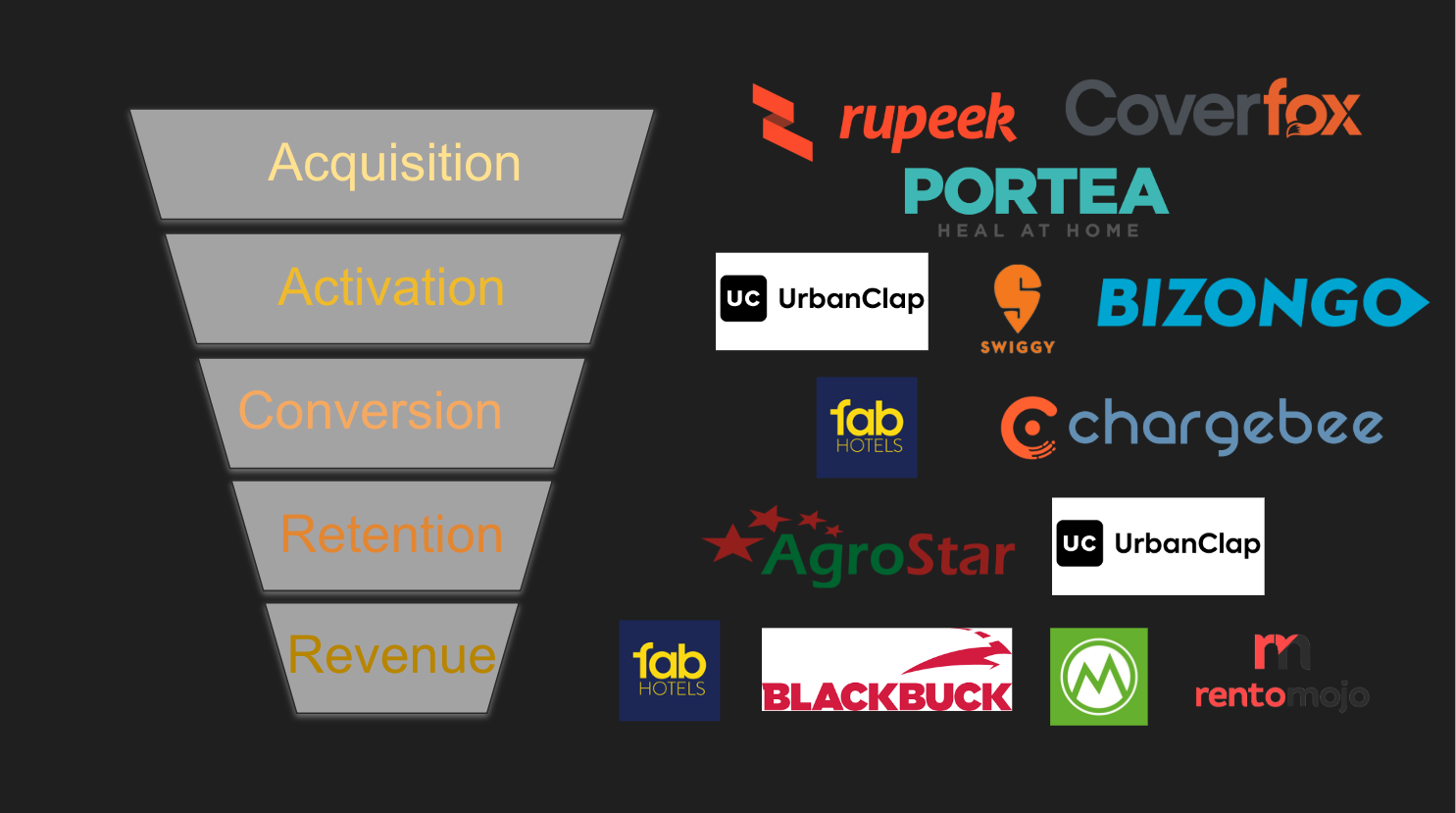
Study of data science use cases across Accel portfolio companies.
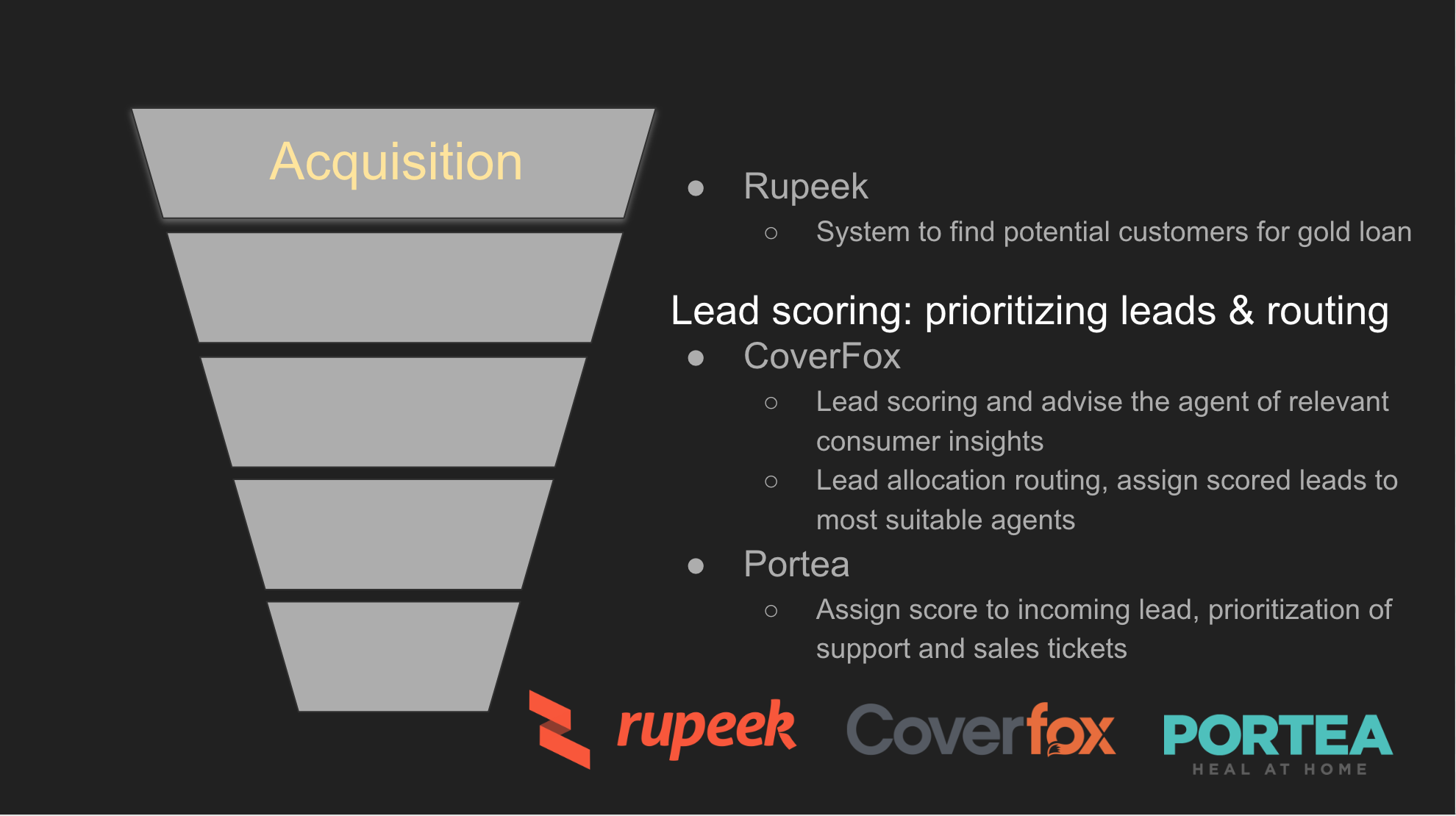
Note: We will take only few use cases to convey the message “benefits of applying data science in existing business problems “ in this blog post.Post your question in the comment section to know more about specific use case .
CoverFox-Lead scoring:
Since we are able to prioritise the leads, most effective leads will be attended first along with 360 degree view of customer to our internal agent.This will help us convert more leads into prospect customers.Sending these leads to most suitable agent will help increase customer satisfaction and minimising ticket time. Also, building lead scoring engines can greatly benefit companies in similar domains.

UrbanClap- Matching Customer Needs to Professionals
Initially, merely key attributes such as location, professional rating, and the category of the service platform were used to match professionals to customer services, but this system left professionals and customers unsatisfied. Thus, tree-based models were developed that accounted for approximately 45 features such as the time of day, work experience, and other factors. This resulted in a marked improvement in satisfaction for both customers and professionals.
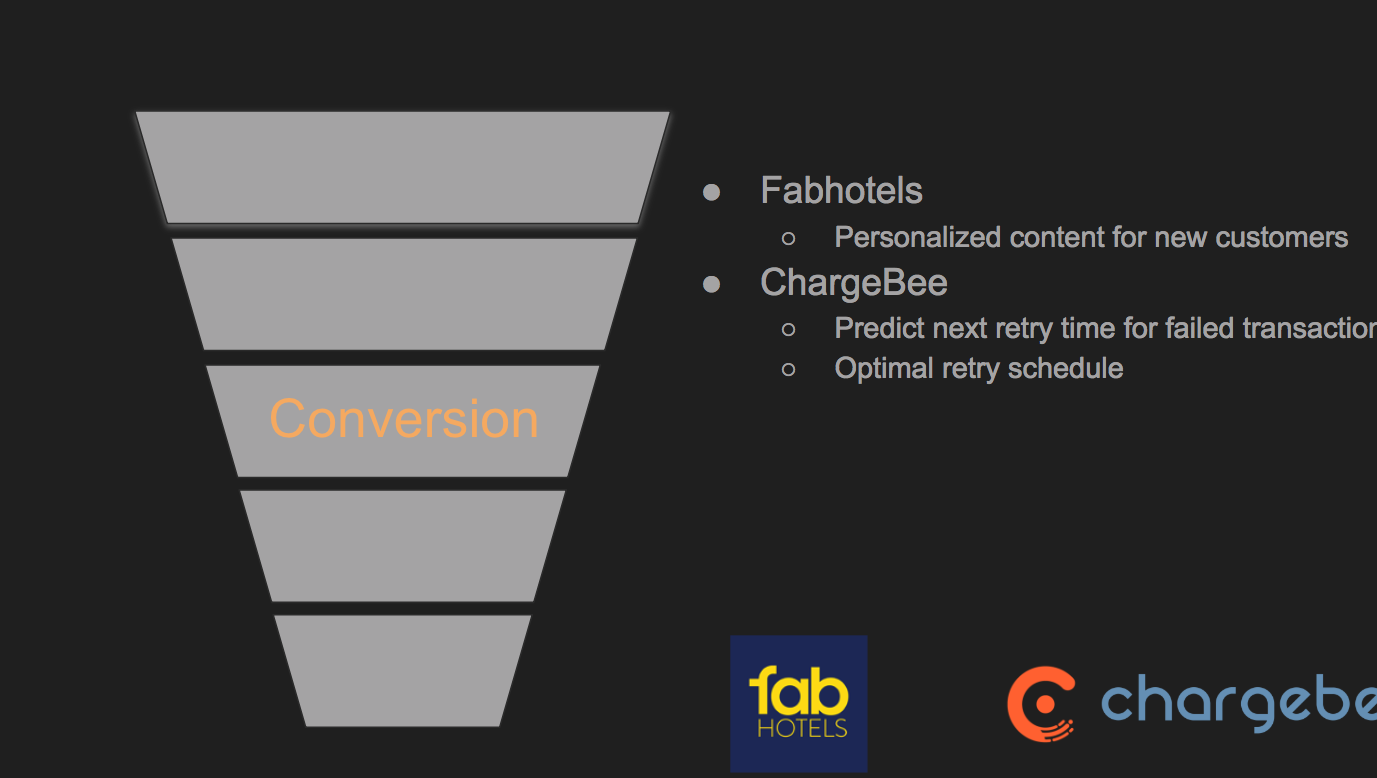
ChargeBee- Optimal retry reschedule :
ChargeBee is a recurring subscription billing SAAS business. For ChargeBee, 5% of all renewal transactions were failing. Thus, the ChargeBee team took to data analysis in order to better discern the reasons behind this decline and if they could identify any apparent trends.
Some errors indicated that the payment gateway configuration needed to be optimised and that requirements for CVV or AVS may not be configured for recurring payments. B2B merchants tend to see errors relating to “SERV NOT ALLOWED” when customers make payments using corporate cards with restrictions on recurring transactions. Likewise, B2C merchants may see this error more frequently when customers make payments with prepaid debit or gift cards.
After establishing the decision-tree-based model, they were able to minimise the rate of declined transactions. In turn, this had a direct impact on revenue and reduced churn for customers.
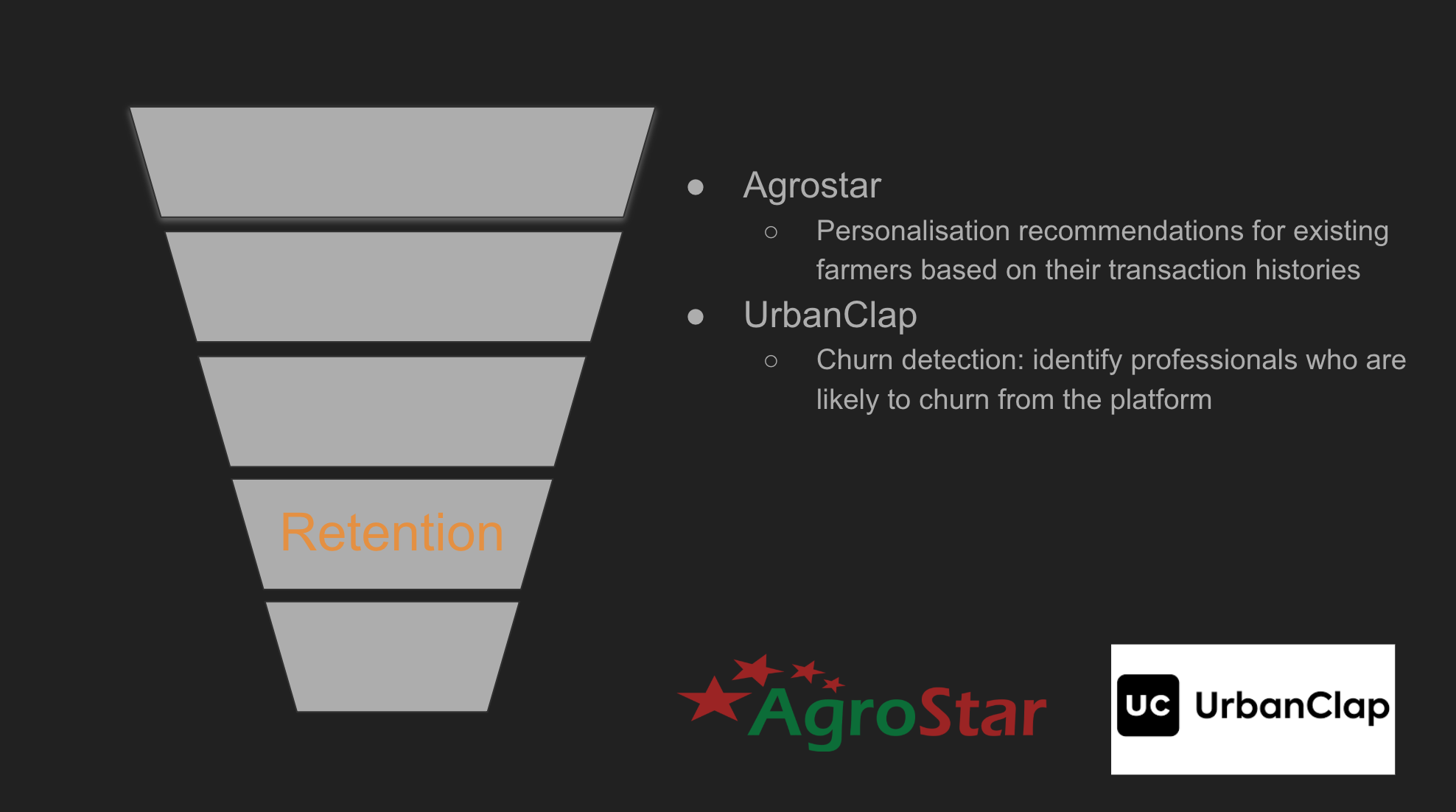
UrbanClap — Churn detection:
Retaining trained professionals is a challenge for any business. For certain categories, such as plumbers, electricians, and the like, professionals are trained by the company itself. Thus, there is high benefit in evading turnover to have access to their continued services. Team has built a basic binary classifier to help predict churn-out within 30 days. Moreover, patterns of churning out are also sought, such as fewer tickets assigned to the individual, no incentives, and so on.This is helping us to minimise professional churn and increasing customer satisfaction(since services are provided by more experienced professionals)
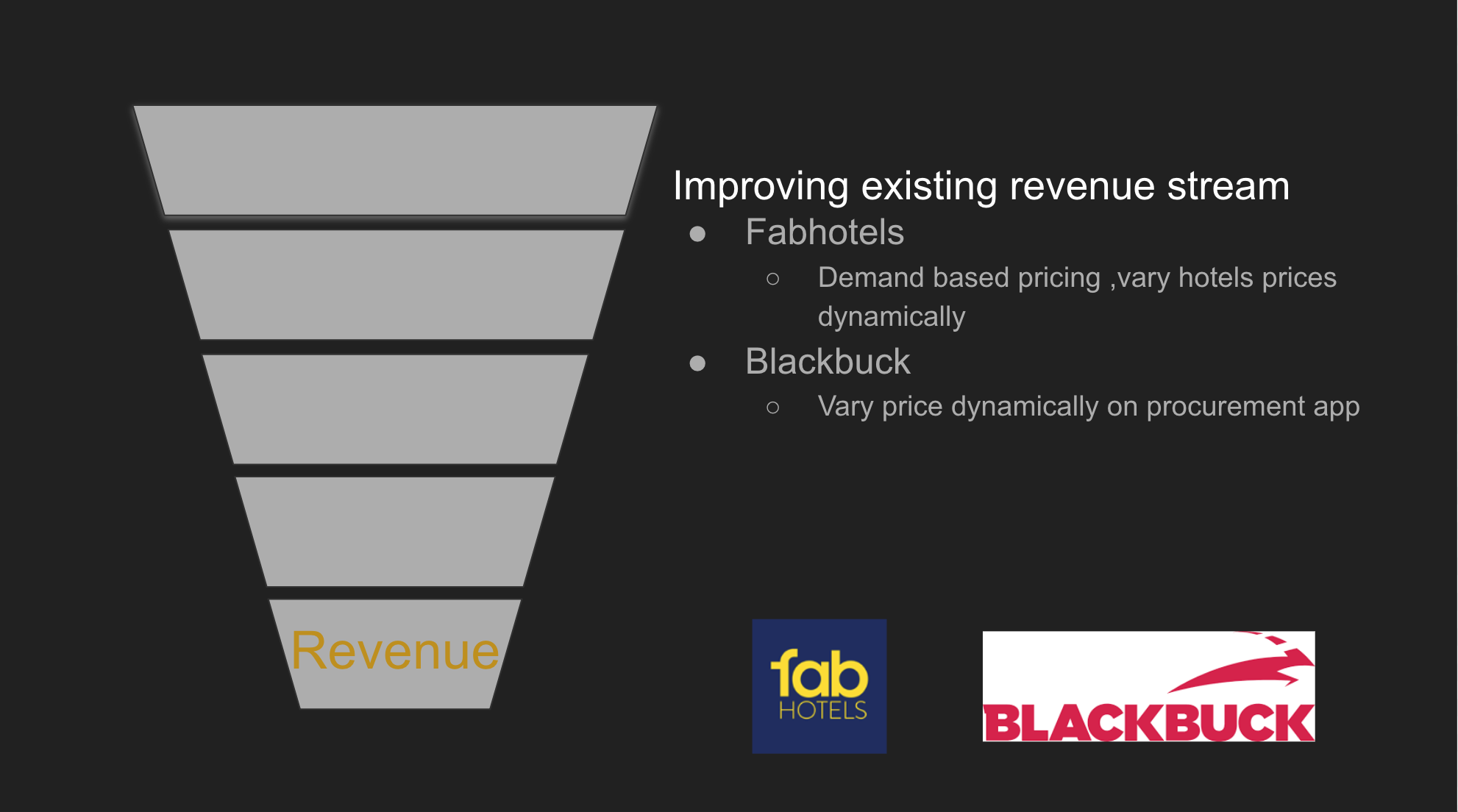
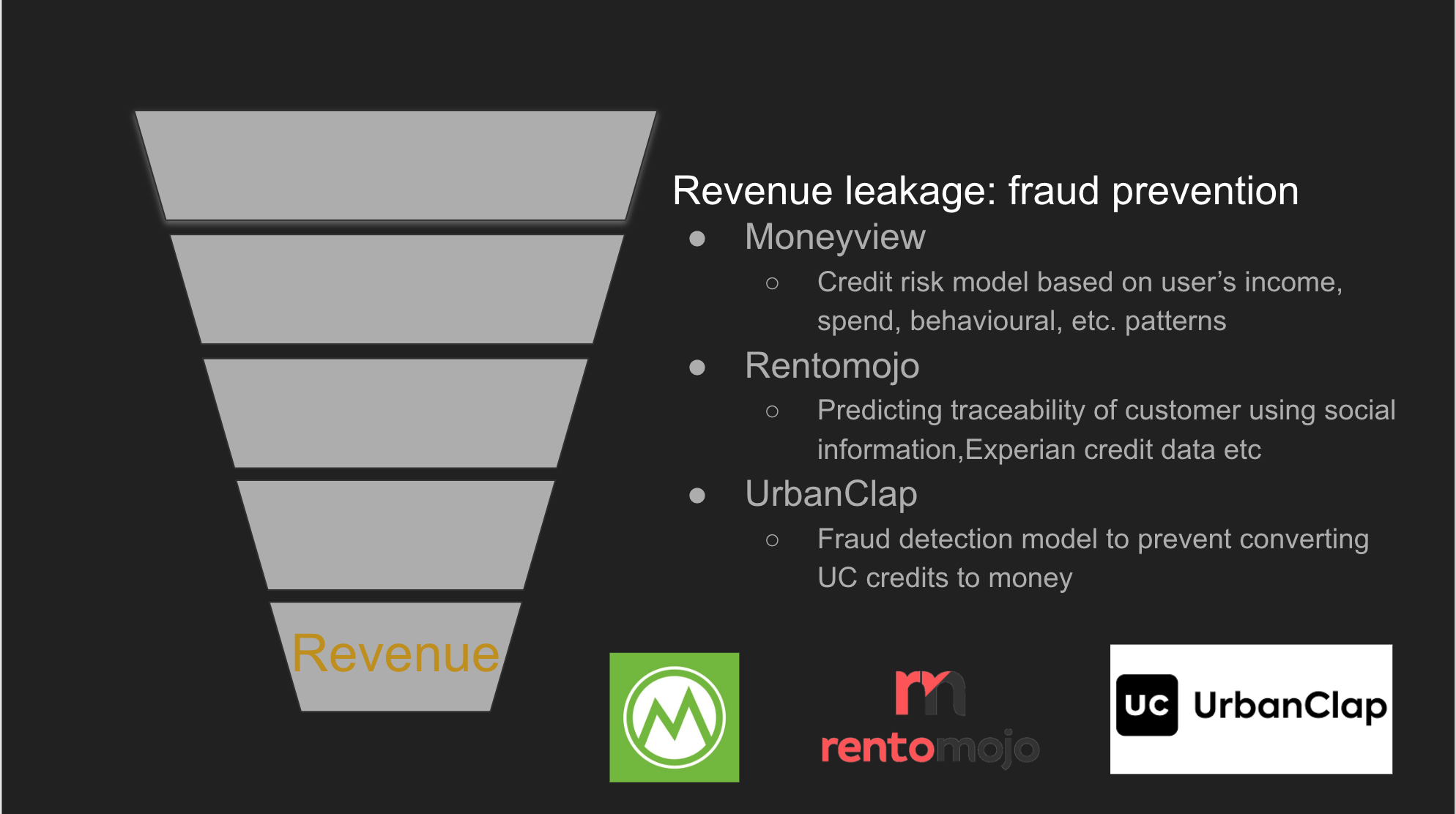
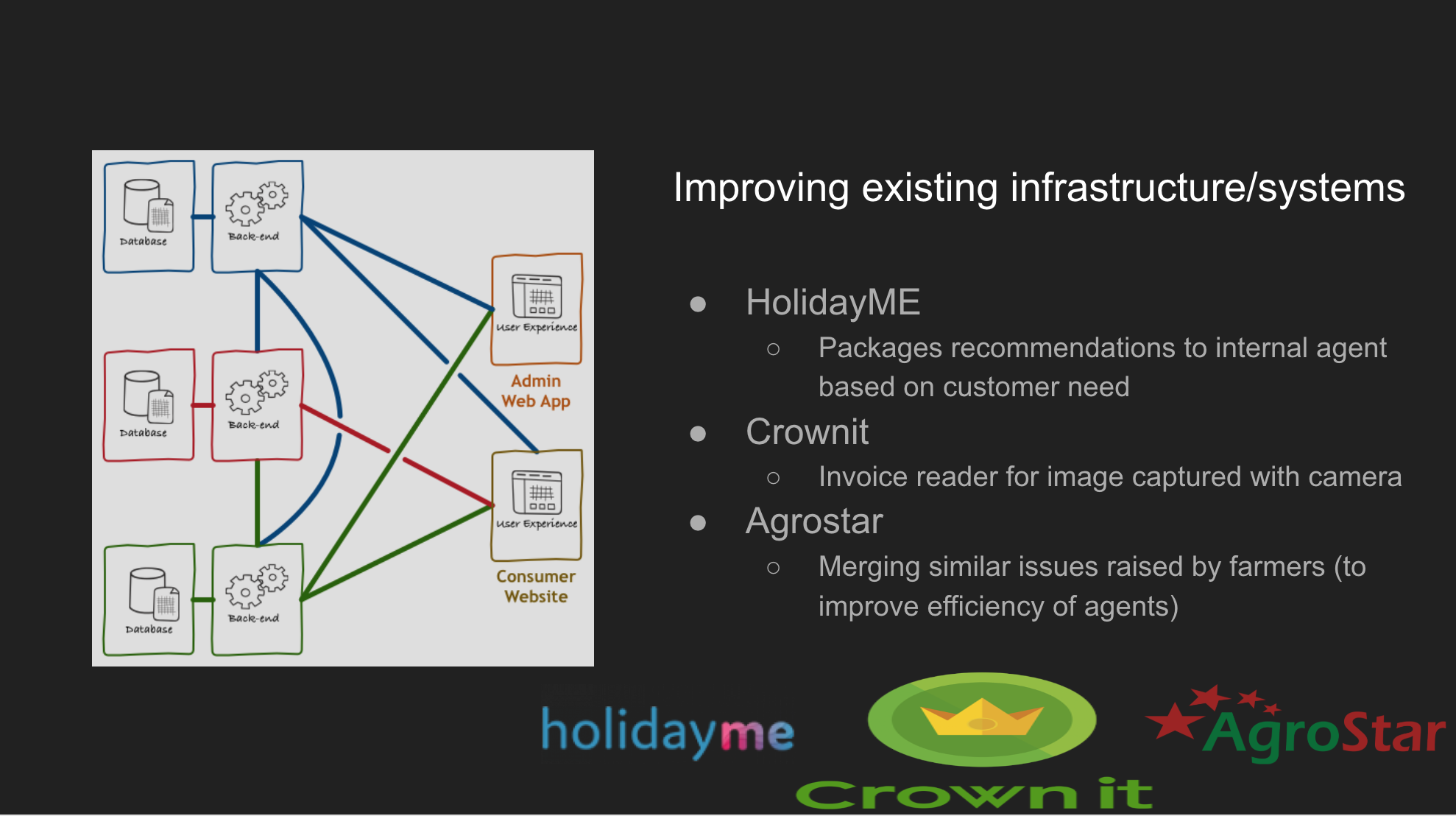
all the problems mentioned above were solved using basic exploratory data analysis ,regression, clustering and tree based models except Crownit -invoice reader → We tried with support vector machine but accuracy was not good enough. Then We built the CNN based model which improved the system accuracy by 10%. As we can observe here almost all the business problems were solved with basic data science techniques if you have enough and structured data in place.
Accel encourages startups to build a strong foundation of data before venturing into the territory of data science, artificial intelligence, or machine learning.
We found Data science “HIERARCHY OF NEEDS” digram very useful.What we will see in media everyday about AI, deep learning which is relatively hard to apply especially if you are business company driven by technology and you don’t have in-house data experts .
NEEDS TRIANGLE [DATA SCIENCE]
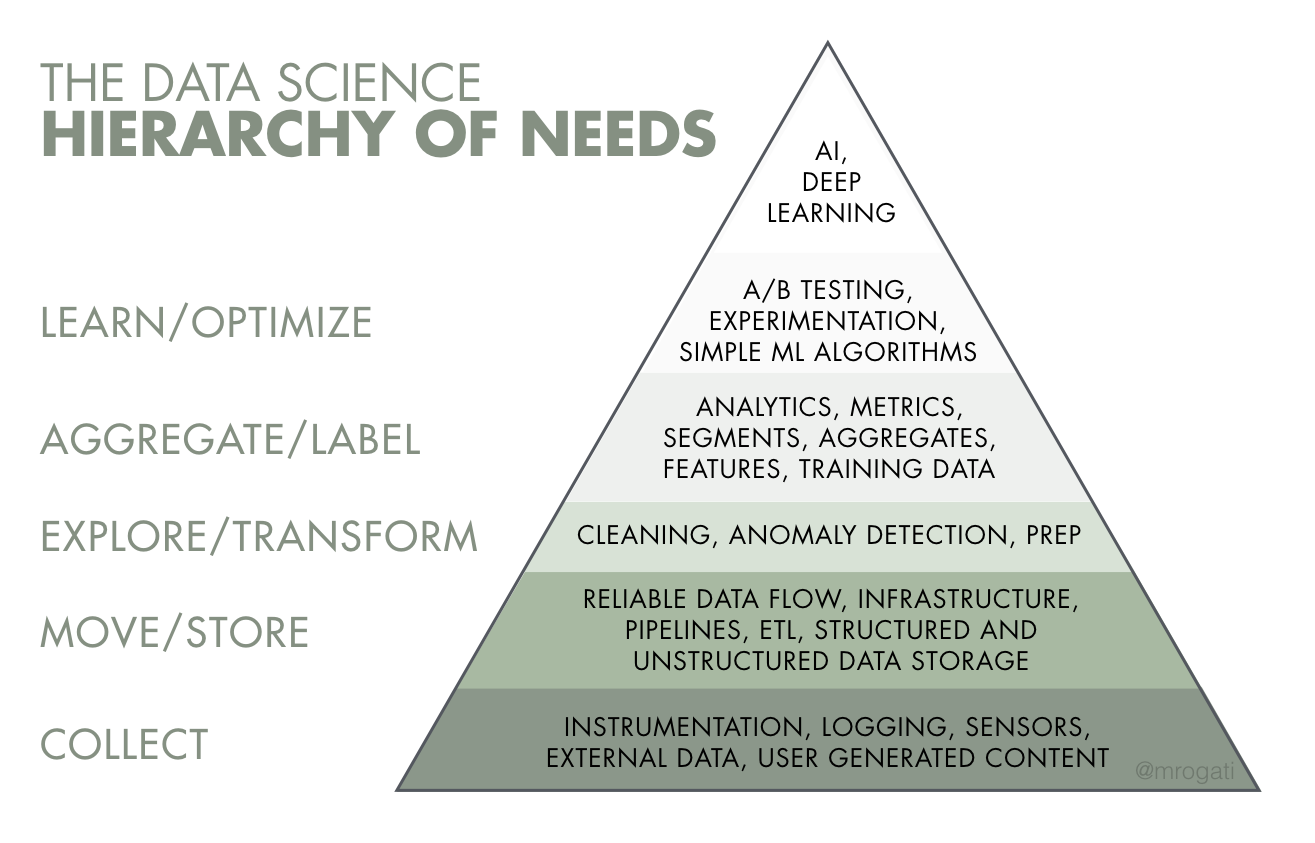
Making AI work for your business [Case studies from Freshworks]

ML is core of the Freshworks Products. Each product line is driven by Data .
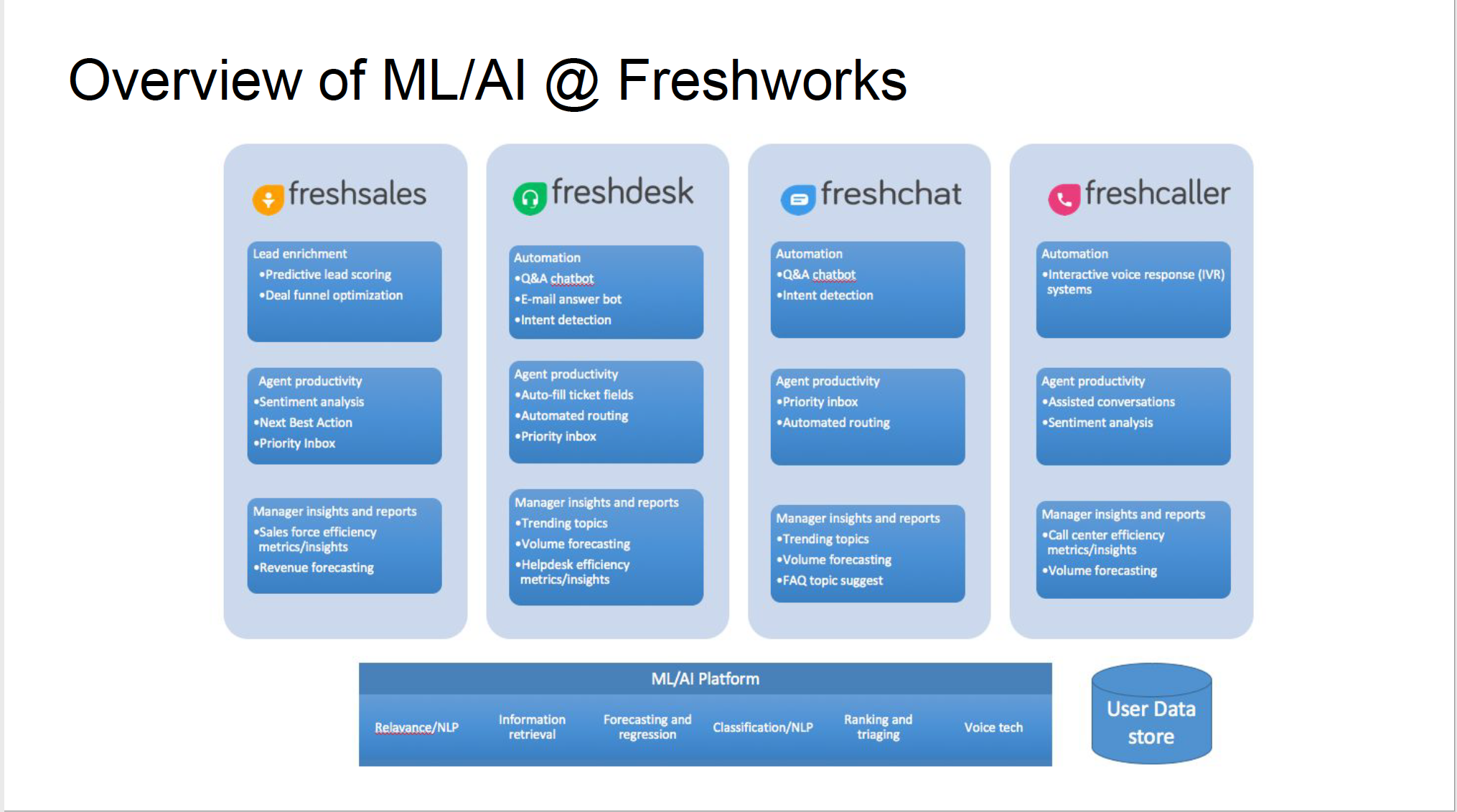
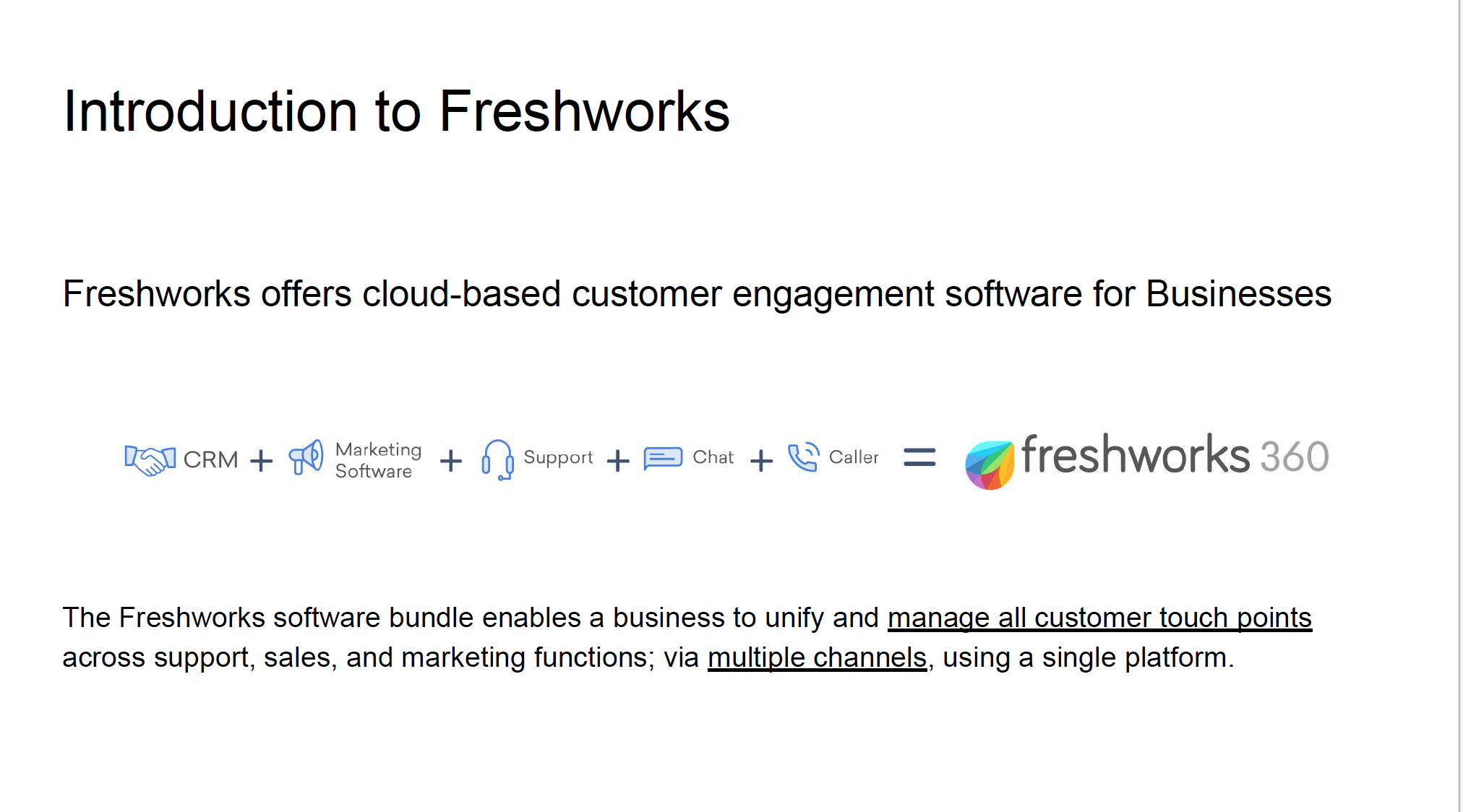
The audience was presented with two important cases wherein data science was used to improve business processes and increase revenue.
1. Incident (ticket deflection in customer support) :
Recently, customers have begun to expect DIY forms of customer service. This is because these are often the fastest and lowest effort ways to resolve problems. There is no need for an external agent when the issue is relatively simple.
For companies, this is beneficial because increasing self-services lead to improved ticket deflection. This is when customers choose to help themselves rather than reach out for support. This allows support teams to focus on more complicated issues. Moreover, since the issue is resolved instantly, customer satisfaction is high.
Simple search engine-based approaches do not succeed here due to lack of domain understanding and contextual information.
For instance:
Q1. Will you give me my money back if I don’t get a service call by Thursday?
The expected answer is a refund policy, but the search engine cannot handle this.
Q2. How much do you charge for a 3-year old?
The expected answer is pricing for children, but a search engine cannot be trained to answer these problems. There exists a definite need for data science in the form of NLP or machine learning tools.
Freshworks uses NLP and machine learning to automate high-frequency, low-touch customer interactions and bypass the effort required for customers to discover content. Whenever the platform is not able to handle support requests, it is passed on to an appropriate agent. This results in great cost-cutting for Freshworks.
A feasibility analysis for this issue was conducted to decipher if enough data was present for analysis. We asked the following questions:
- How many customers have a knowledge base? (Most do)
- What percentage of queries can be answered using the customer’s existing knowledge base?
- Per model for each customer? (Industry level models)
- Apart from knowledge base, what else can be learnt from tickets, previous chats, forums, and the like?
- How do we incentivise customers to grow their knowledge base? (This is a very critical matrix because when a more expansive knowledge base is added into the platform, the model becomes more accurate.)
After evaluating such data verticals, enough data was found to apply data science to this use case.
An ML platform was built for incident deflection and assisted resolution, which provided customers with the following services through this platform:
- Chatbots
- Email answerbots
- Similar incidents
- compose assist
- social bots
- Collaborator assist
Results:
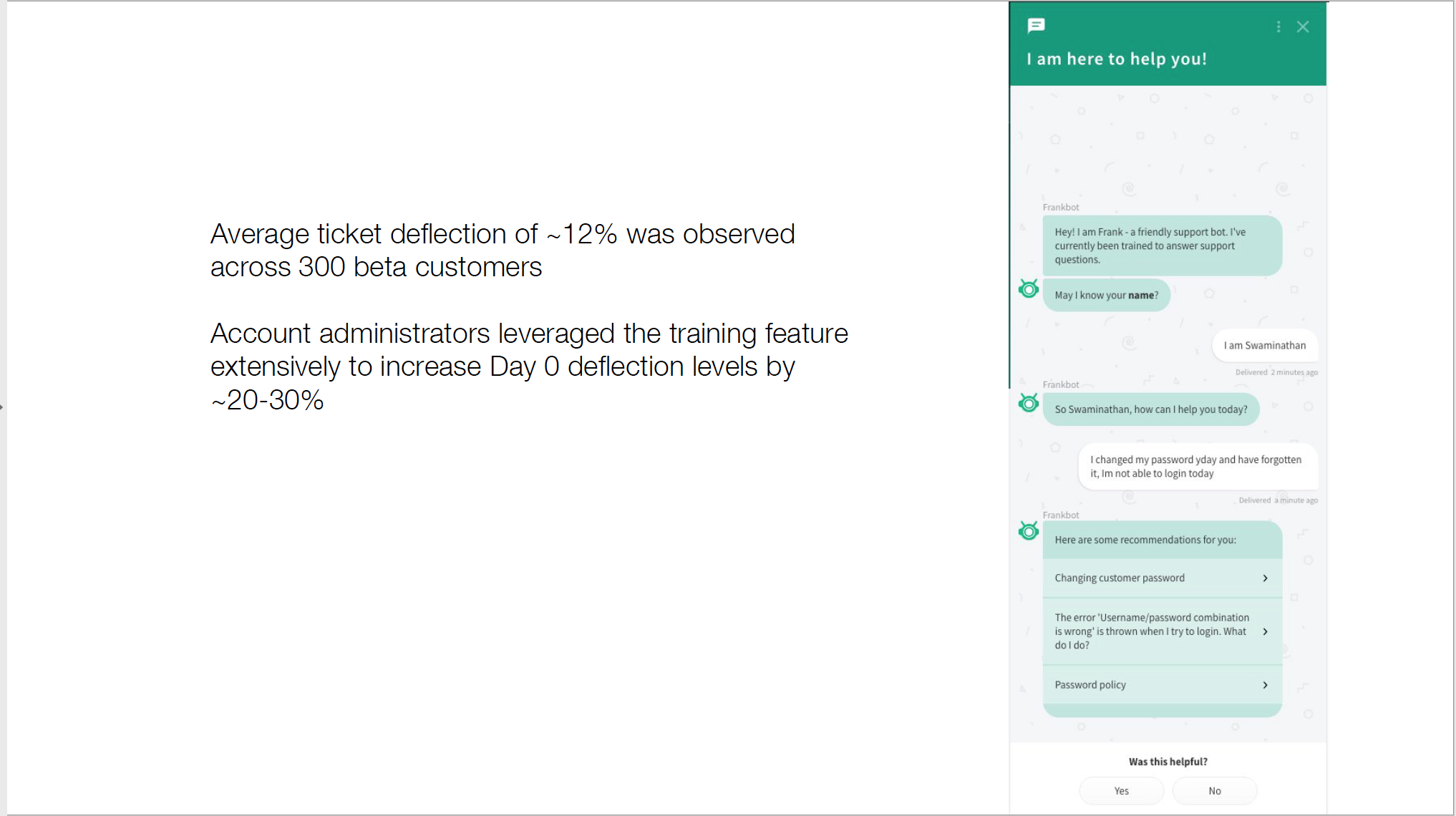
2. Predictive deal scoring :
The Goal: We will want to prioritise which accounts we reach out to.
Assigning a score for all inbound leads based on how likely they are to convert (as a customer is the essence of lead scoring) is predictive deal scoring. The below problems were worked on using this technique:
- Sales teams typically have a large number of open leads in their pipeline
- In conventional CRM, there is no predictability on which deals would close and by when
- There is no tools available to help a sales agent determine how to accelerate deal closure
A feasibility analysis using existing data was performed to check whether a deal is predictable or not.
“A good predictive model needs good quality data in large amounts!” by Swami
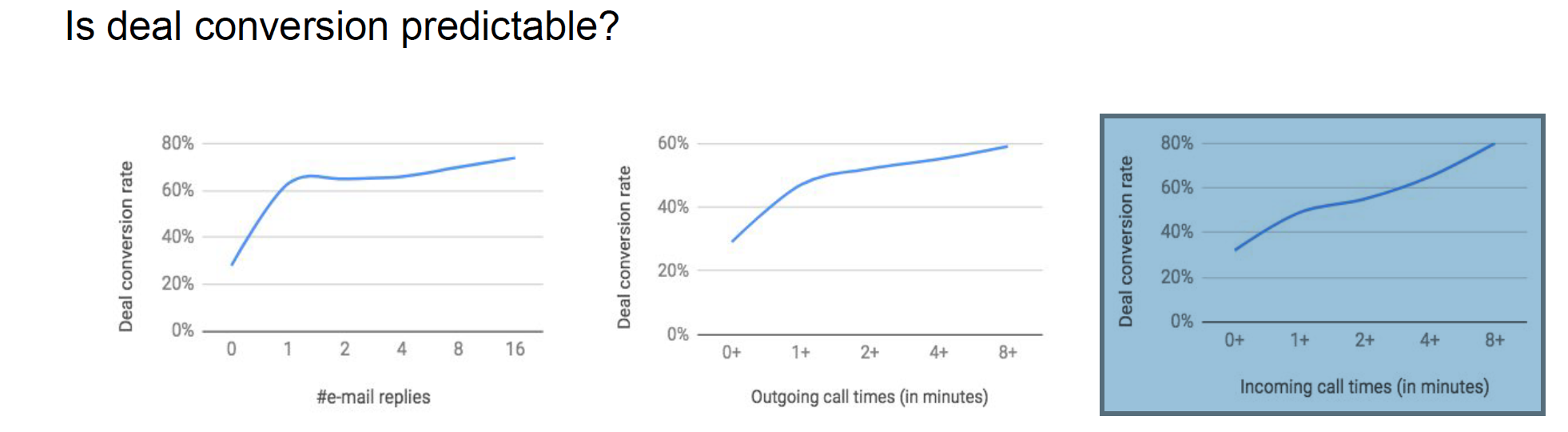
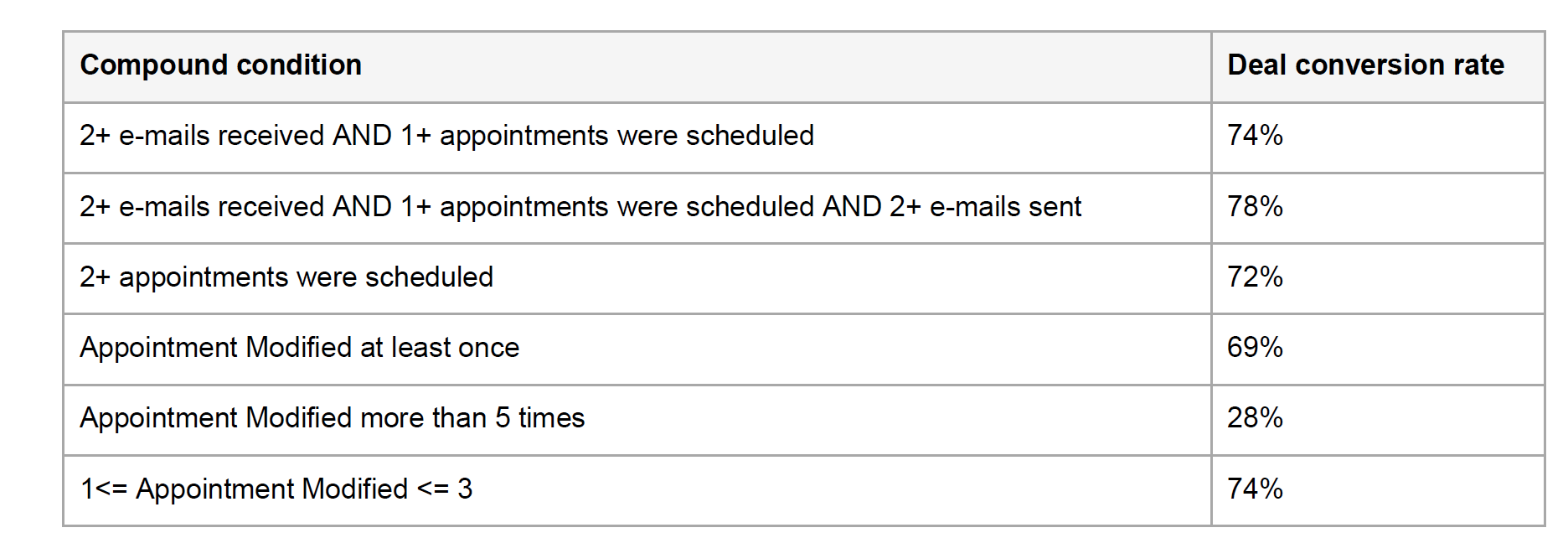
From the above diagrams, one can discern that the lead scoring engine could surely be built. The regression model was used to achieve this.
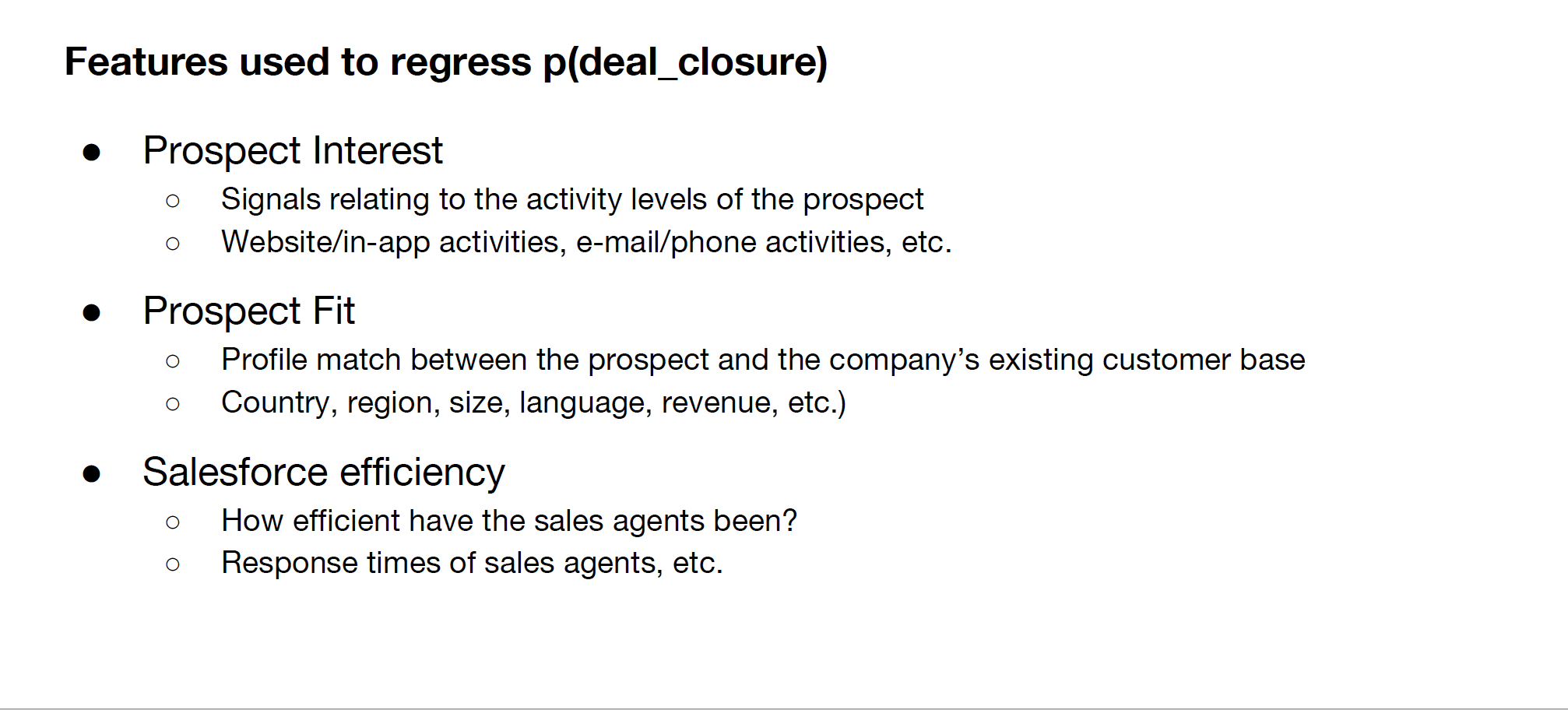
There may occur situations where the data is not sufficient enough to build deal scoring systems. For example, when new customers and industry level cases are involved. In such scenarios, fallback logic is employed wherein models are trained at an account level.
There were cases where CRM data was incomplete on account of low fill rates due to erroneous filling by the sales team. In such cases, other attributes such as revenue, web traffic, and industry, among others, are used to predict lead score.
Results:
- Predictive lead scoring enabled the sales team to focus on the best prospective leads and optimise conversions
- Beta experiments indicated that sales teams could isolate around X% of the conversion by focusing solely only on Y% to Z% of their total outstanding leads. Where X,Y,Z are some percentage of impact.
Data Science - what is it good for?
[Case studies from Myntra]

“Data is gold, You need a solid foundation for your data before being effective with AI and machine learning ” Ambarish
Identifying Fashion trends:
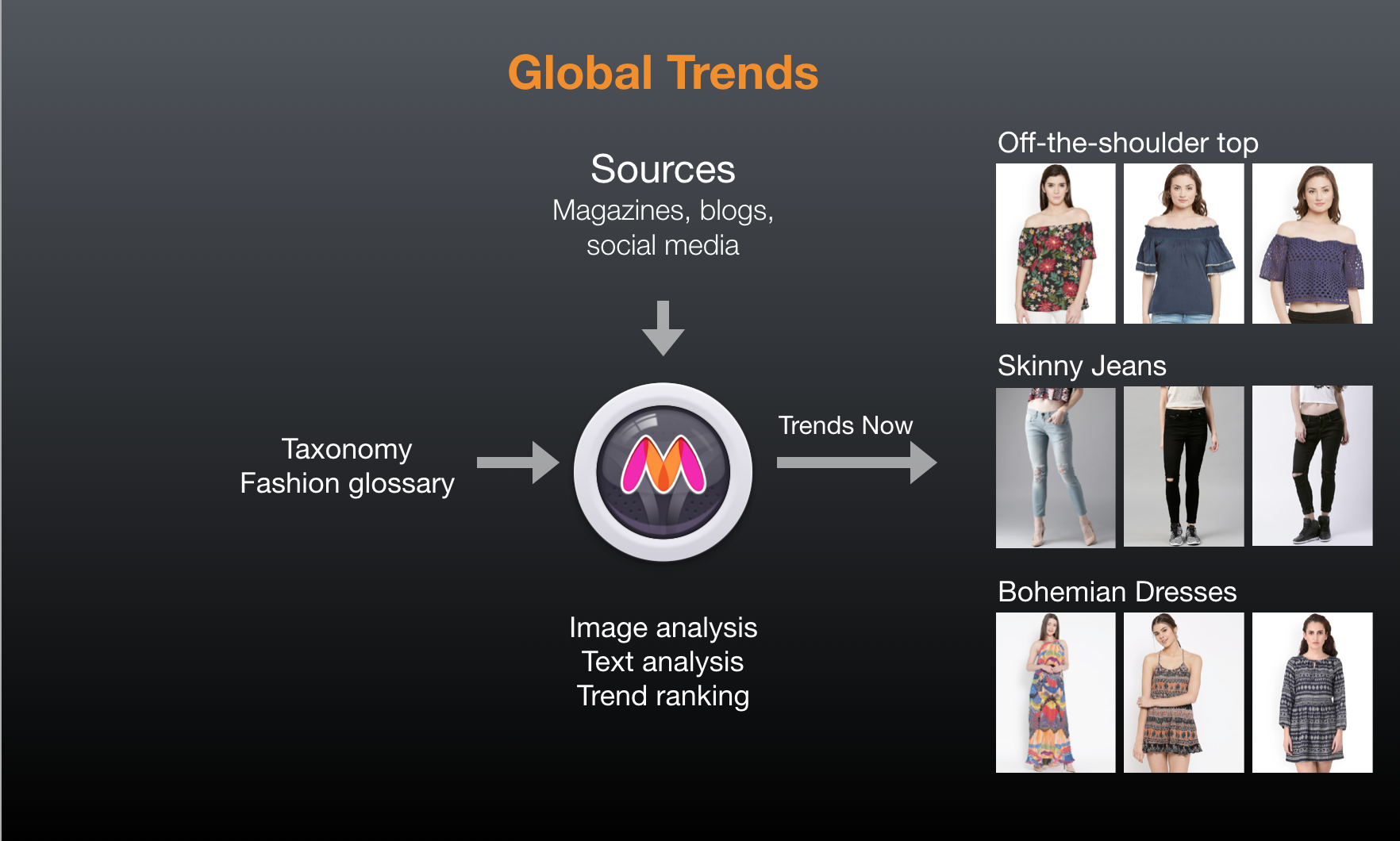
Results:
- Revenue impact per impression
- CTR went up
Personalisation:
Myntra emphasises the value they place on data in order to tailor recommendations, engage influencers. and customise experiences. Consumers are willing to share data if it provides a more personalised experience online. Fashion trends today are fleeting, which is why discovery is very important for better engagement and sales revenue.
Initially you don’t need to build complicated system, start personalising, from where user left in last session something like recently viewed items once user re- login to platform. And then build collaborating filtering , this approach really worked out for Myntra. Iterating fast and running experimentation was the key for this system.
Personalisation appears easy on the surface, but for a company with Myntra’s scale, it does become challenging. The process of personalising from thousands of brands, millions of products with different sizes, and the failure to predict the correct sizes for personalised items, leads to frustrated customers.
One way to build size recommendations is to study the customer’s purchased and returned items. Data on customer returns enable the building of a system to recommend sizes. Data collection across multiple levels is therefore critical.
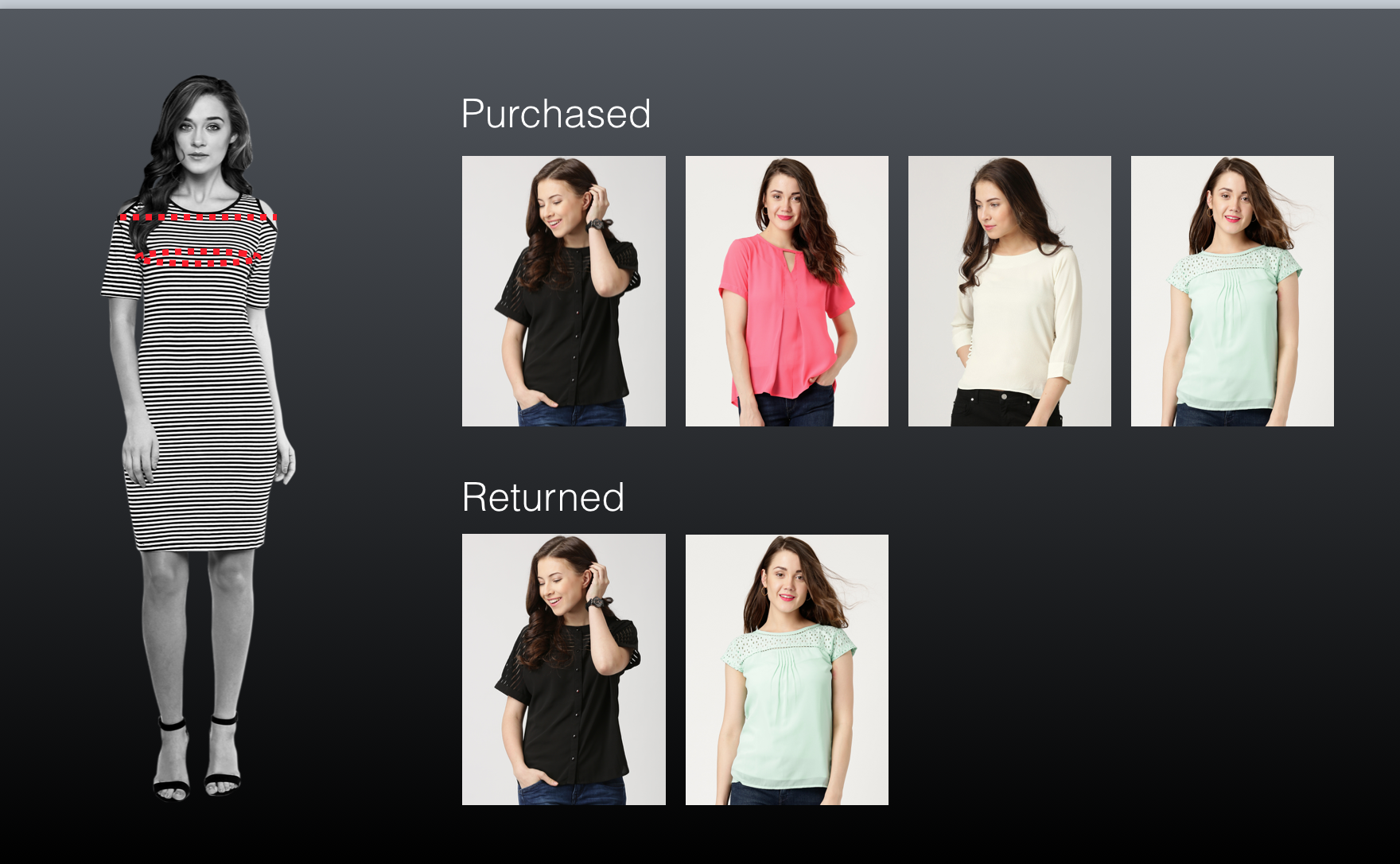
Another scenario is, “What if I am ordering this purchase for someone else?”
This is where data strategy enters. One could enquire from customers, “Who did you order this for?” And use this data to further personalisation.
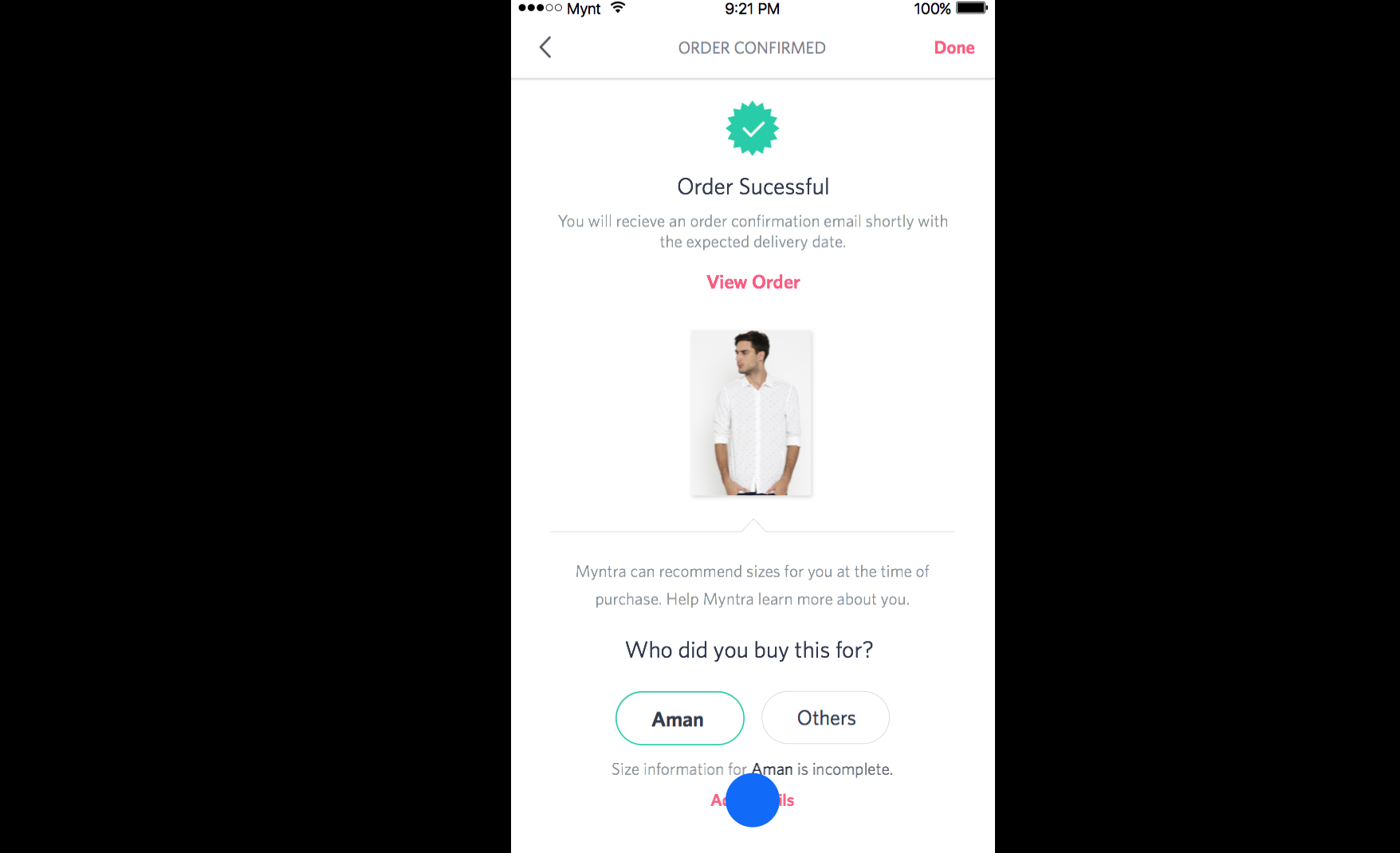
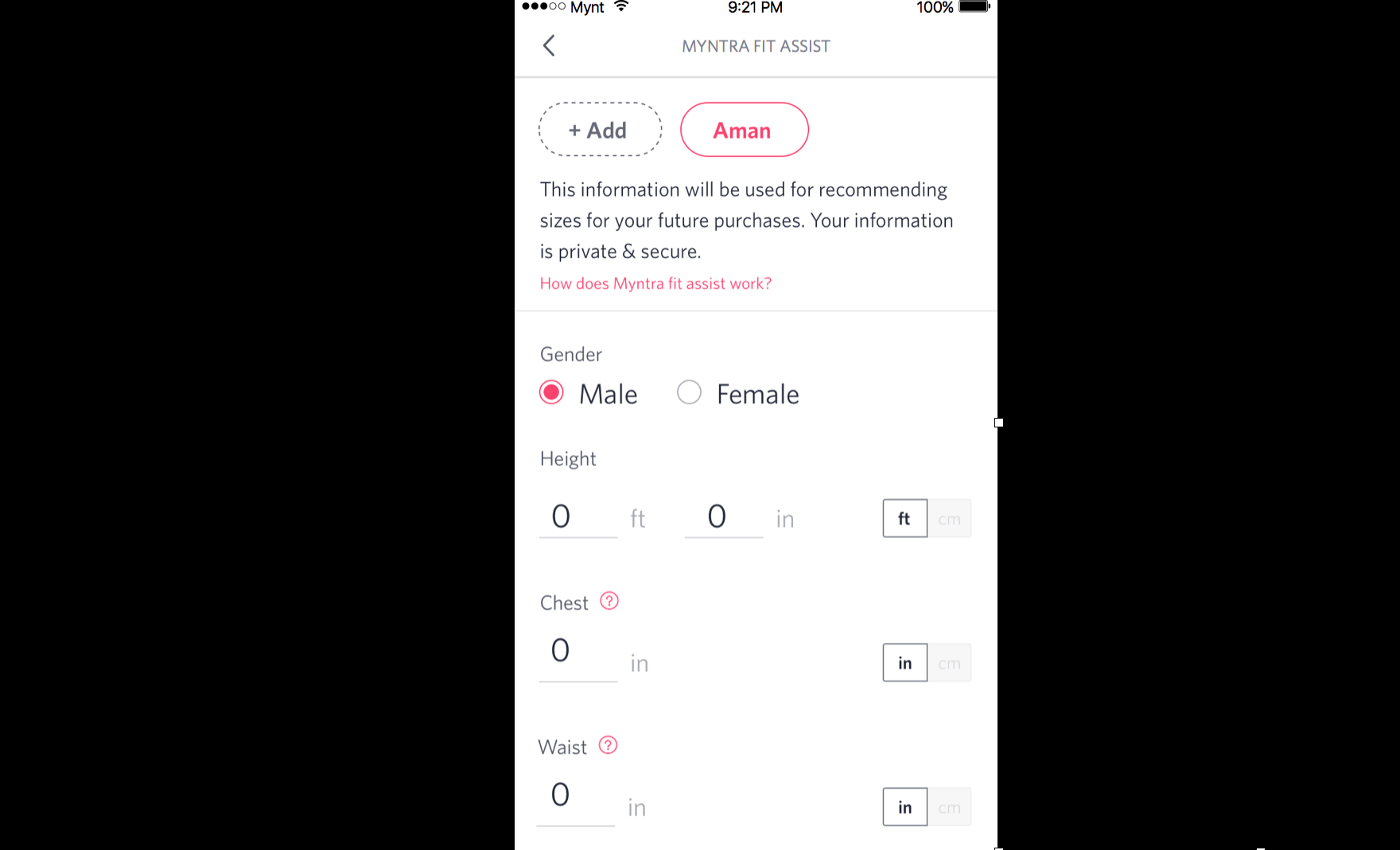
Myntra has run various experiments to design data collection strategies for different sets of customers. It benefits to reward customers in order to accrue this data.
Results:
- CTR increased for personalised banners compared to equivalent no personalised banners
- X% of increase in CTR
- Personalised sort order for ~ Y million users
Search:
In the beginning, general sentiments of search were not optimal. There were several areas where customers were not provided with meaningful results. Information retrieval systems such as Elastic search failed to comprehend queries such as “casual shoes under 400.”
“why, many queries where we have not provided intended result or provided no result”. Data from the following verticals were used to answer this:
- query log
- Feedback system from customer care
- A Myntra-wide bug bash to collect feedback
Problems from this experiment were clustered into three areas:
- Failure to find intent or context of the query “casual shoes under 400” should be understood as the customer searching for casual shoes with a limit of Rs. 400. “Moto 360” must be understood as “Smart wearable ” and “Fog” must be understood as “deodorant” in order to suggest customer-appropriate products.
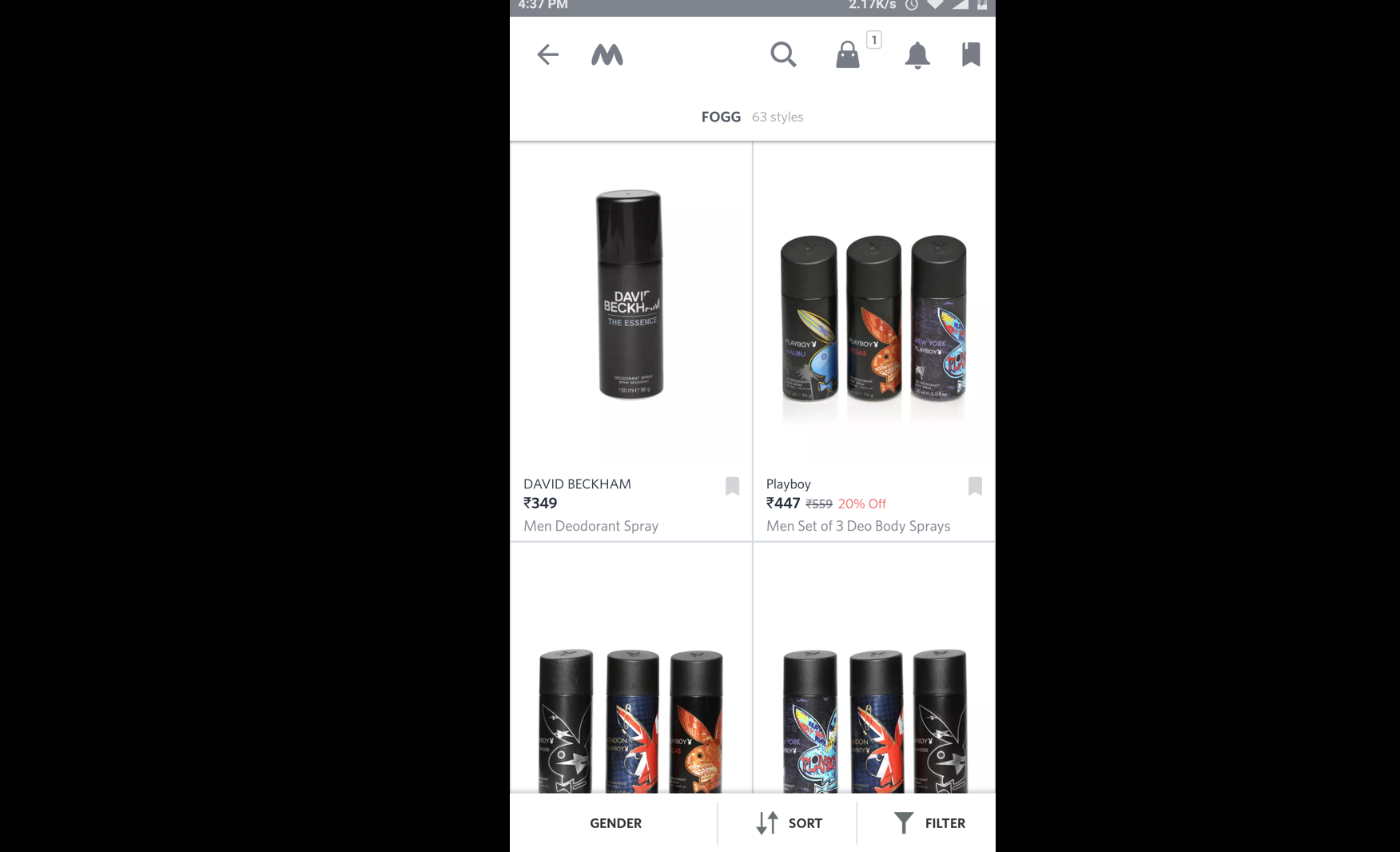
- Spell correction did not seem to correct common scenarios like “jins” to “jeans.”
- No query substitutions were available. In the case of no results a probable substitution was not offered.
It is evident from the above results that the problem relates to either precision or recall and since both cannot be solved simultaneously, the choice was made to improve precision. For this, the click-through rate was researched. The bounce rate, click-depth, and zero results were found to be most important.
Based on this research, the below pipeline was built:

This resulted in fruitful engagement and an increase in revenue.
Cross sell:
- Expert tell is what to cross sell
- data science collaborative filtering approach increased the CTR by X% .
Myntra’s Intelligent fast Fashion -> Rapid Platform [example of AI] relatively hard science problem.
That’s Myntra Fast Fashion for you — fashion via high-tech engineering.
This platform is the perfect example of using the already collected data and insights to create new revenue stream and value for customers.
Myntra Fast Fashion entails fashion via high-tech engineering. The production processes deliver the latest trends in the market, which usually span 6 months. These were reduced to under 30 days.
“In the initial days, there was less machine and more designer input. With rapid platform, we utilised more machine input and less designer supervision.” — Ambarish

Myntra uses social and various other data sources to sense demand and global trends. The sales data across Jabong, Myntra, Flipkart were included to help develop a machine-generated design.
Results:
Two brands launched using Rapid platform generated huge demand among customers.
The Rapid Platform is an example of intensive data science and a more sophisticated AI.
Takeaways:
- Make data easily available to data scientist
- Build for experimentation
- Cross function team
- Beware of spurious models and correlations
A Q&A with our experts:
Which should be hired first at a startup: a data engineer or a data scientist?
Ambarish answers: Definitely a data engineer, making the data scientist your first data hire is the common mistake startups make. Unless you already have a solid data infrastructure and internal business intelligence (BI) practice, you’ll need a data engineer to build pipelines and help data scientists prepare data to prevent boredom and turnover. If you hire a data scientist first, they won’t have any data to play around with. We did this when we hired our first data scientist. He work for 6 months to bring the data into the appropriate format and only then started solving data science problems. Hiring a data engineer reduces the scope of work for the data scientist because data prep steps can be handled by data engineers. Get an experienced practitioner for your first data hire; this guy will be able to move quickly with minimal assistance, which means you will see faster returns on your data science investment.
How do you develop a data road map, that is, a data strategy?
Jeet answers: Data can be used to drive decisions and build products that increase profits, reduce costs, reduce risks, engage customers, boost operations, and generate insights. Develop a set of questions you’d want to answer, connect “what we want to do” with “how will we do it.”
Participate in the survey here to understand where your startup stands in terms of a data roadmap.
Conclusion, key takeaways:
- Define your first data project in detail:
- What questions are you trying to answer?
- Will these improve the business matrices? - Map problems to existing data. If you have enough data, start with simple data science techniques to solve immediate important cases and then delve into more advanced techniques like neural nets.
- Data matters even to startups. If you are not processing data for insights during your early days, archive the raw data in some form of storage such as S3.
- Focus first on Low hanging fruit — Least adopted “must do” use cases, for example for ecommerce industry Forecasting, Tracking consumer behaviour , reducing revenue churn. For insurance industry lead scoring, cross selling etc. All these problems can be solved using simple technique like regression, multi-class classification , tree based models etc.
- The domain of data science is experimental. Invest and build a culture of experimentation and fast iteration for the data science team. Work in small iterations based on results and learnings.
- Craft a clear vision for what the company wants to achieve with data science, explore basic data science ,high value use cases and simple data science technologies to implement them first.
- Launch Proof of concepts for selected use cases and run them in staging environment before pushing them in production.
- Set up a cross-functional team for each data science use case; horizontal teams produce better results for data science projects.
- Hire experienced data engineers before hiring data scientists. Look for experience in the data scientists you hire. Entry level candidates may be slower on account of having to learn processes. Experienced practitioners will be able to move faster and manage teams.
For future blogs on data science please subscribe to Accel India or follow here.
Related Articles:
We’re looking for the next generation of successful Indian marketplaces. If you’re an early-stage marketplace founder, apply now here or learn more about the #DecodingMarketplaces Startup Hunt.
We’d love to hear about your experiences with marketplaces. Let us share our learnings and build a better and stronger ecosystem. Write to us at seedtoscale@accel.com to be a part of the Accel family.
Subscribe to SeedToScale
/subscribe to get the latest stories from SeedToScale/